TL;DR
- Reading machine-translated articles resulted in lower synchrony in brain activities across people in the brain region “precuneus“, which has been associated with social cognition.
- This is one of the first studies applying “natural stimuli” and “inter-subject correlation” to study the neuroscience of reading.
- This study was published with the title “Differential brain mechanisms during reading human vs. machine translated fiction and news texts” on Scientific Reports (Co-first-authored with my Master’s degree advisor)
- Components of this study were presented at the following conferences as posters:
- 2015 Organization of Human Brain Mapping
- 2017 Society for Neuroscience
- I won the Third Place in 2015 National Taiwan University Three Minute Thesis by recounting this study.
Google translate is a helpful tool, but the results can be misleading… especially before 2016
Have you ever used Google Translate? When we have a basic level of understanding for some foreign language, but have trouble understanding a particular sentence, Google Translate may hint at the structure of the sentence. The result churned out by Google Translate may serve as the reference based on which we may piece together the real meaning of the sentence. Yet, we all well know that the machine-generated text can’t be the ground truth, our own discretion is still required.
After the introduction of the neural network technology in 2016, the translation suggested by Google Translate (let’s call it GT for short, since it will be mentioned repeatedly in this article) became ever more precise and human-like. However, it still yields error from time to time, especially when:
- A sentence is taken out of its context
- A particular concept doesn’t exist in the target language
- The same sentence is to be translated into multiple target languages
Here’s an example of a sentence which could make GT go awry, taken from a manga I have been reading for a while. The context of the sentence is that the invincible main character was asked by her girl friends about self-defense moves. And she said:
護身ったって、そんなカラまれるコトないからな
This sentence can be roughly translated as “Self-defense move, huh? Well… I’ve never been caught up in such a situation (that I’d need self-defense), though.” But what GT yields is “Even if you defend yourself, you can’t get caught like that.” (as of 2023 Apr. 30th) Japanese is highly context-dependent, to fully understand a sentence, we have to consider when it is spoken, and WHY it is spoken — that is, we have to speculate the intention of the speaker. GT lacks the information about the context and the ability to conjure one, thus yielding bizarre translations like this one.
Things get even more absurd when we ask GT to translate the same sentence into another language, say Traditional Chinese. Here it goes, “即使你為自己辯護,你也不能這樣被抓住“, which basically means “Even if you defend yourself, you can’t get caught like that.” But the sense of the word “defend” in the Chinese translation is “to act as attorney for” rather than “to drive danger or attack away from” as in the original sentence. Apparently, when I asked GT to translate a Japanese sentence to Chinese, it first translated the sentence to English, then it translated the English sentence to Chinese, losing track of the original meaning — about which GT had no idea in the first place.
GT finds the statistical relationship between words within the same language or even across languages, but it has no idea about what this relationship means.
That’s why we sometimes find GT results hilarious. In fact, before 2016, the results generated by the old version of GT were almost always hilarious. The sentences were passable, the meaning was more or less conveyed, but the wordings and arrangements were just outright weird. While reading the sentences, we could almost hear a robot speaking a language it can barely grasp. It was just awkward.
In my Master’s degree study, I looked into the manifestation of this awkwardness in our brains.
Readability: an emergent property of texts
We might all agree that texts generated by GT is not particularly readable, but it’s not that easy to articulate what factors contribute to the “readability” of a text. While I’m writing this article, a “Readability analysis” panel to the right of my WordPress editor was keeping track of the readability index of my writing. It suggested that the following features may lead to a low readability:
- Containing a lot of hard or unusual words
- Containing a lot of sentences in passive voice
- Containing a lot of long sentences/paragraphs/sections
- Lacking variability in the way sentences begins
- Not using enough transition words
Obviously, none of these is the problem of any single word or sentence. A rare word like “floccinaucinihilipilification” is just that, rare. We wouldn’t say that this word has low readability. The same roughly goes with sentences. A single sentence in passive voice? No problem. A single long sentence? Probably fine. We wouldn’t consider a passive sentence or a long sentence particularly unreadable. But yeah, if a long sentence is also convoluted in clause structure, we’d say it’s not very readable. Like this example sentence I found while searching for “long and convoluted sentences”:
The life story to be told of any creative worker is therefore by its very nature, by its diversion of purpose and its qualified success, by its grotesque transitions from sublimation to base necessity and its pervasive stress towards flight, a comedy.
Note that the words in this sentence are not outrageously hard, but the way they got strung together leads to a complicated sentence. So, basically,
Readability is an “emergent” property that lies not in any single element, but in how these elements are combined.
Therefore, to study the neural effect of readability, I can’t use individual words or sentences as our experiment material. Instead, I adopted whole articles which people may encounter in everyday life. In other words, I conducted my experiment with “natural stimuli” (more about natural stimuli can be found here)
Reading, as naturally as possible during an functional magnetic resonance imaging (fMRI) scan
In this experiment, I looked at not only the effect of readability (resulting from different sources of translation), but also the genre of the texts — fiction vs article. Consequently, each of my participants read four types of articles:
- Human-translated fictions
- Machine-translated fictions
- Human-translated news
- Machine-translated news
The articles were chosen from well-accepted English publications with reliable official Traditional Chinese translations. Namely, fictional articles were selected from Reader’s Digest, and news articles were selected from The New York Times. The original (English) version were fed to the then pre-neural-network GT to generate machine-translated versions (FYI, this study was done during 2014-15).
Here are some examples of the English version, the human-translated version, and the machine-translated version of a fictional article and a news article. For simplicity, I only show the first paragraph of each article. If you happen to know Traditional Chinese, you can experience firsthand the difference between human and machine translations.
Fictional article (English): “Where Frogs Quack”
It was one of the weirdest bits of news I’ve ever had to break. “Something unusual is infesting the earth under our apartment block,” I told my neighbor. “I think it’s a herd of cows.” Below our building and the field nearby could be heard the unmistakable moo of large cattle. It went on for days. Yet none of my encyclopedias, nor that trusty compiler of oddities, the internet, had any information on “burrowing cows”. The closest I could get was “ground beef”.
Fictional article (Human-translated): “青蛙都是「呱呱呱」?”
現在想起來,下面是我跟別人說過最詭異的事:「我覺得在我們的公寓底下有些不尋常的東西,」我對鄰居說:「我覺得是一群牛。」在我們樓底下和周邊的田裡,可以清楚聽見只有大牲口才會發出的哞哞 聲。這種情況持續了好幾天。不管是在我那些林林總總的百科全書裡,還是網際網路這個最可信賴的奇聞異事搜尋工具那兒,我都沒能找到關於「地下穴居牛」的資訊,我所能找到最接近的是「碎牛肉」。
Fictional article (Machine-translated): “青蛙都是「呱呱呱」?”
這是新聞,我曾經不得不分享最古怪的之一。「一些不尋常的為害我們的公寓樓在地下,」我告訴我的鄰居:「我認為這是一群奶牛。」下面我們的建設和現場附近區塊可以聽到大牛的哞哞無誤。這個狀況持續了幾天。然而,沒有我的任何百科全書,也不是那個值得信賴的奇怪的現象編輯者,互聯網,有對「穴居牛」的任何信息。最近我得到的是「碎牛肉」。
News article (English): “Creatures That Hide in the Ocean”
The oceans, which make up more than 90 percent of the earth’s livable space, are full of almost invisible animals.
To illustrate why, Dr. Sonke Johnsen, a professor of biology at Duke University in North Carolina, began a recent talk with a macabre scenario. Suppose just then a gunman burst into the room, shooting at the audience. Naturally, people would scramble for cover behind chairs and walls.
His point: There would be places to try to hide.
News article (Human-translated): “海洋動物藏身於無掩蔽處”
海洋占地球可居住空間的90%以上,其中充滿各種幾乎不可見的生物。
為了證明箇中原理,美國北卡羅來納州杜克大學生物學教授江森最近以一種駭人的場景展開對話。假設一名持槍男子闖進這個房間,向著聽眾開槍;人們當然會躲在椅子與牆壁後尋求掩護。
他的論點:總會有那麼些地方可以躲藏。
News article (Machine-translated): “海洋動物藏身於無掩蔽處”
海洋,從而彌補了超過90%的地球上的可以居住空間,是充滿了幾乎看不見的動物。
為了說明為什麼這樣,江森博士,生物學杜克大學在北卡羅萊納州的教授江森,開始了透過一個可怕的場景在他的會談。假設就在這時,一名持槍男子衝進房間,在觀眾射擊。當然,人們會爭奪搶背後的椅子和牆壁覆蓋。
他的觀點是:將會是有的地方,可以試圖隱瞞。
During the experiment, participants silently read such passages during an fMRI scan. While the articles were natural reading materials, I didn’t want my participants to read completely naturally so as to involve a lot of regressions (re-reading previous sentences), which might result in drastically different reading time across participants. Therefore, I designed a scrolling reading interface which constrained participants’ field of vision to the current line plus or minus 2 lines.

This design ensured participants are generally reading the same part of the article at the same time, enabling the analysis adopted for this experiment.
Inter-subject correlation revealed the neural difference between translation styles and genres
If a bunch of people experienced the same sequence of events at the same pace, at least somewhere in their brains, the neural response time-course should be very similar. For example, when different people listen to the same song, the time-course (or waveform) in their primary auditory cortex should be almost the same — thus highly correlated. That’s because the primary auditory cortex more or less faithfully represents incoming audio signals, that is, the song.
This is the idea underlying the “inter-subject correlation (ISC)” analysis.
In so-called “higher-order” regions in the brain, the neural time-courses are not replications of the perceived events. Instead, they may track the feelings, interpretations, or predictions for the events a person is experiencing. Because feelings, interpretations, and predictions can vary across individuals, how correlated the time-courses are between people may reflect how universal the response to an experience is. If a story is very explicit and obviously happy, we may observe high inter-subject correlation in these higher-order regions. On the contrary, if a story is ambiguous, the inter-subject correlation value can be low. More about inter-subject correlation and related analysis methods can be found here.
I applied this inter-subject correlation analysis to the neuroimaging data collected from natural reading. My hypothesis is that there should be a difference in the degree of inter-subject correlation between different translation styles, and between different genres.
And such a difference I found.
Human-translated articles yielded greater synchrony between readers than machine-translated articles. This effect resided in the right brain, in a region called the “precuneus“, which has been associated with social cognition, or the ability to imagine other people’s mental states.
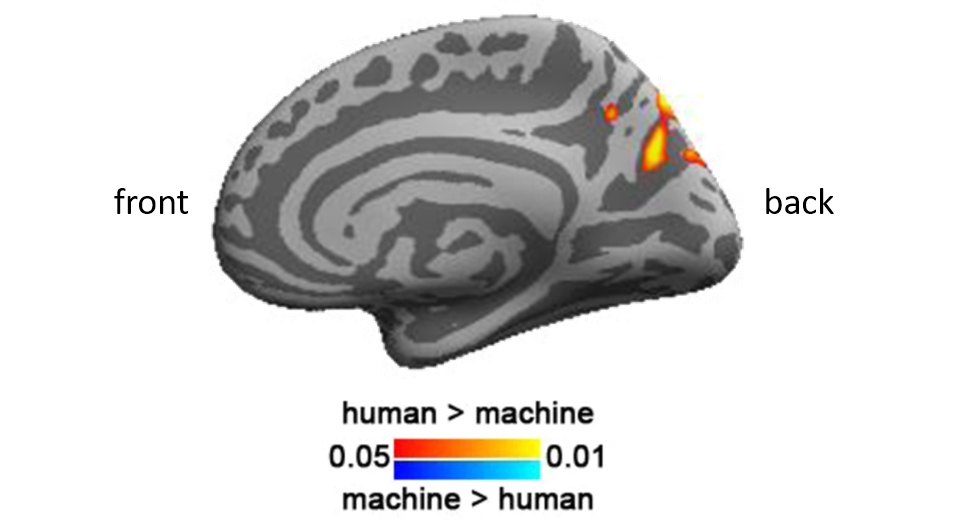
So, I probably found the neural/mental effect of the low-readability characteristic of machine translations: it gives people a hard time understanding the mental states of the characters involved, or coming up with some thoughts of their own regarding the events described by the articles. Even when the articles are really simple, people just couldn’t understand them in the same way.
As for the difference between genres, I found greater inter-subject correlation for fictional articles than news articles. This is again in accordance with common wisdom, though the effect of the difference lies in not in the precuneus, but is found mostly in the primary visual cortex (which faithfully reflect the visual input) and the anterior temporal cortex (temporal pole), which is usually associated with the integration of meanings during reading. As for what this indicates, interested readers are welcome to read my published work.
Re-visiting this study done in 2014 after so many years (it’s 2022 when I wrote this), I found much to improve regarding the design and analysis method. But to my knowledge, this work is still among the first ones to apply “natural stimuli” and “inter-subject correlation” techniques to study reading. Thus, I’m still proud of this work, and thankful to my Master’s thesis advisor, Dr. Fa-Hsuan Lin for letting me play with these ideas previously unheard of in the lab.
Related scientific publications
- Lin, F.-H., Liu, Y.-F., Lee, H.-J., Chang, C. H. C., Jaaskelainen, I. P., Yeh, J.-N., & Kuo, W.-J. (2019). Differential brain mechanisms during reading human vs. machine translated fiction and news texts. Scientific reports
- Hasson, U., Nir, Y., Levy, I., Fuhrmann, G., & Malach, R. (2004). Intersubject Synchronization of Cortical Activity During Natural Vision. Science
- Hasson, U., Malach, R., & Heeger, D. J. (2010). Reliability of cortical activity during natural stimulation. Trends in Cognitive Sciences
- Honey, C. J., Thompson, C. R., Lerner, Y., & Hasson, U. (2012). Not Lost in Translation: Neural Responses Shared Across Languages. Journal of Neuroscience
- Bedny, M., Pascual-Leone, A., & Saxe, R. R. (2009). Growing up blind does not change the neural bases of Theory of Mind. Proceedings of the National Academy of Sciences